More than two billion people purchased goods or services online in 2021 with sales exceeded $4.9 trillion globally, according to Statista. We know what these people purchased but cannot predict with certainty what they will want to buy in the future. With the invention of new technology, such as machine learning one can collect and analyze user data more accurately. This makes it easier for companies to research and discover emerging trends, and use this knowledge to adjust their marketing strategy. This is called trend scouting, or demand forecasting. In this post, we will look at the process and its three main stages.
The ability to analyze large sets of data has always been crucial to a business’s success. It used to be done manually, with large groups of people. Now, however, there are computers and neural networks to do this work for us. Since the process has been automated, it happens faster, meaning that much larger data sets can be processed. Why analyze this data and how does it work?
The idea behind this approach is to “have computers make sense of textual content produced by people,” according to Andrey Nekhaenko, Retail & Agriculture Lead at DataArt. Having analyzed this content, computers can then recognize and categorize the patterns they find. The content is primarily sourced from social media, but also comes from internal information systems and databases.
The news should be analyzed as well, as it might provide important information about the market and existing trends. This analysis helps companies understand how a specific trend was formed, how we can expect it to develop, who was it formed by, and its messaging. At DataArt, we use business intelligence methods to transform data into these insights.
This analysis is also sometimes called natural-language understanding. It is essentially a way to understand textual meaning, and this technique has been around for a while. Automation has changed natural-language processing in several ways, including speeding up the process. Previously, data scientists would first come up with a hypothesis and then test it. Now, we collect these data sets first, hoping they will somehow inform our decision making.
These insights can indeed be quite informative. Brands use them to identify their target audience and map out user journeys. Based on this analysis, PR managers and marketers can come up with more efficient ways to connect with their clientele.
Retailers need natural-language analysis to decide what products to store, to see what sells, and to decide what to put in catalogues. It also is a great tool for retailers to strike a better deal with their suppliers. Once you know what sells best, you also know what sells poorly.
This helps you negotiate discounts for the products that may have strong competitors. This is an example of how demand forecasting lets you track competitor behavior and navigate the market to drive your business. So, how do you forecast demand?
A great way to uncover new trends is to track patents. New patents are registered every day, and it’s hard to track them manually due to the sheer quantity of data. With automation, companies use algorithms which sort through these patents automatically and categorize them by industry/field. By doing this, one can locate specific patents that interest them and get a sense of what tendencies exist and what people are working toward.
Tobacco companies are an interesting example of this. When Philip Morris introduced the IQOS line of electronic cigarettes in 2014, they patented it. There were no analogical products on the market at the time. If their competitors were tracking this development, they would realize the significance of the product and could start developing their own. If they did, IQOS wouldn’t dominate the market and something like GLO, a similar product from British American Tobacco, would have come out sooner. This principle can be applied to any market.
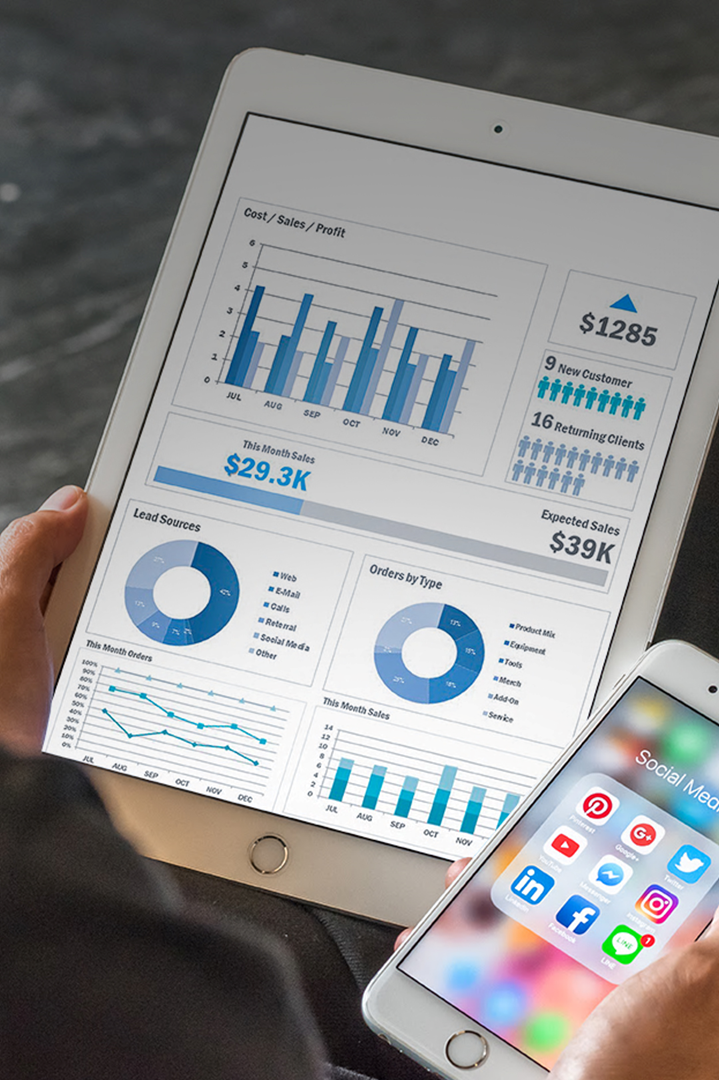
Another great way to find useful insights is by looking at social media. Some brands focus on online channels and rely on influencers for promotions. They understand their target audience by looking at these influencers’ followings. These brands can then gather analytics and decide which influencers to launch their products, depending on what their audience is. This is a way to improve marketing tools and target specific groups. This kind of demand forecasting allows us to identify audience parameters, their interests, and communication preferences.
The trend scouting process can be broken into three main stages. During the first stage, information needs to be gathered. The second stage is when this information is sorted — it has to be categorized and anything irrelevant should be removed, which requires to develop some algorithms internally. The third and final stage is processing. Data is processed using machine learning, which helps classify and analyze texts. Based on the results, companies determine what needs to be accentuated/reworked in their texts and strategy. But what technologies are used to gather information?
Data is usually collected by algorithms that draw it from a variety sources. If there is an application programming interface (API) or a specific database you are looking at, you can use a connector to get data from there. If it’s social media, the companies that operate the sites may be reluctant to share information. Due to user privacy regulations this process isn’t simple and often even impossible, although there are many other open sources where the relevant data can be taken.
Some social media applications have a more accessible API, which allows you to extract information from there. Data can also be easily retrieved from news websites since they let others share and upload their content. This also depends on geographical location. For instance, in some eastern countries, from a legal viewpoint it is not seen as personal data, but in Europe or the US it’s much stricter.
As part of the process, you have to work with data that wasn’t prepared for this use. If it’s a social media post, there could be typos, unnecessary details, emojis, or pictures you have to get rid of. You will also have to introduce word tokenization to split large samples of text into smaller units. For example, if there are different versions of the same word (if the word is conjugated, for instance), you need only the infinitive form. You have to design filters to do this work and to format texts to feed them to algorithms later.
After data sets are ready, the third stage begins. In this stage, you use mathematical methods like clusterization to identify texts/information grouped around the same trend. Trends can be in turn segmented into sub trends. Then, you might form 10-20 sentences that describe the trend. These sentences reveal how it was formed — who wrote these texts, shared or liked them online and on what platform, if there were any positives/negatives, and how people reacted in general.
Some information cannot be categorized or isn’t needed, like topics that everyone talks about — these topics include weather, children, money, and health. Sometimes there isn’t enough information about something to identify a trend at that moment.
An important aspect of trend scouting is that it tends to be collected in a personalized way for everyone. All companies have their own preferences for how they want this data to be collected and sorted. To succeed, this mechanism also has to be able to teach itself, to evolve. The more data – the more precise is the analysis. When you start working on it, you need to know exactly what your goal is, what you want to end up with, and what you don’t want there. It is key to remember that machine learning and AI isn’t magic, it’s math. The quality of your data will rely on how accurate your algorithms were. It might take around 6-8 months to create an efficient trend scouting tool.
Not everybody uses trend scouting, however. Small start-ups, for example, already have a solid understanding of who they sell to, so they don’t always need this kind of research. If, however, a company works with many different audiences, then they most likely need to employ demand forecasting methods.
Nowadays, with so much new information is uploaded and posted daily, trend scouting algorithms play an essential role. If you need professional consultation on how to design and implement these tools, reach out to us!
About DataArt
DataArt is a global software engineering firm that takes a uniquely human approach to solving problems. As a trusted partner with the world’s leading brands, DataArt applies its vast technology expertise and domain knowledge to develop landmark applications.
Powered by our People First principle, we work with clients at any scale and on any platform, helping unleash technology innovation. We develop new products and improve businesses by optimizing processes, improving outdated technology, and creating efficient custom software systems.